
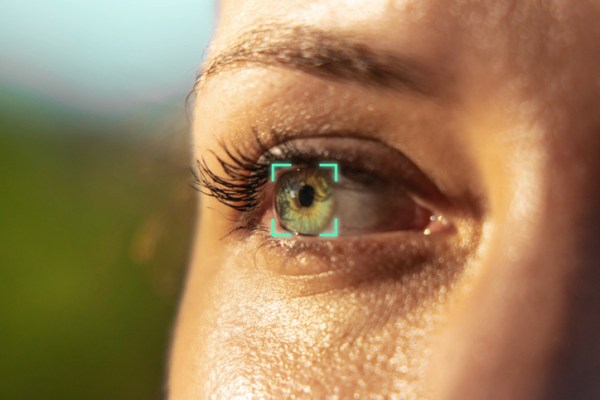
Since they started leveraging the technology, tech companies have received numerous accusations regarding the unethical use of artificial intelligence.
One example comes from Alphabet’s Google, which created a hate speech-detection algorithm that assigned higher “toxicity scores” to the speech of African Americans than their white counterparts. Researchers at the University of Washington analyzed databases of thousands of tweets deemed “offensive” or “hateful” by the algorithm and found that black-aligned English was more likely to be labeled as hate speech.
This is one of countless instances of bias emerging from AI algorithms. Understandably, these issues have generated a lot of attention. Conversations on ethics and bias have been one of the top themes in AI in the recent past.
Organizations and actors across industries are engaging in research to eliminate bias through fairness, accountability transparency and ethics (FATE). Yet, research that is solely focused on model architecture and engineering is bound to yield limited results. So, how can you address this?
Resolving misconceptions on fighting AI bias
Fixing the model is insufficient, as that’s not where the root cause lies. To find out which measures can yield better results, we must first understand the real reasons. We can then look at potential solutions by studying what we do in the real world to tackle such biases.
AI models learn by studying patterns and identifying insights from historical data. But human history (and our present) is far from perfect. So, it’s no surprise that these models end up mimicking and amplifying the biases that lie in the data used to train them.
This is fairly clear to all of us. But, how do we handle such inherent bias in our world?
We inject bias to fight bias. When we feel that a community or segment of the population could be disadvantaged, we avoid basing our conclusion solely on past instances. At times, we go a step further and make inclusions to provide opportunity to such segments. This is a small step to reversing the trend.
This is the very step that we must take while teaching models. So, how do we inject human bias to fight the inherent “learned” bias of models? Here are some steps to achieve that.