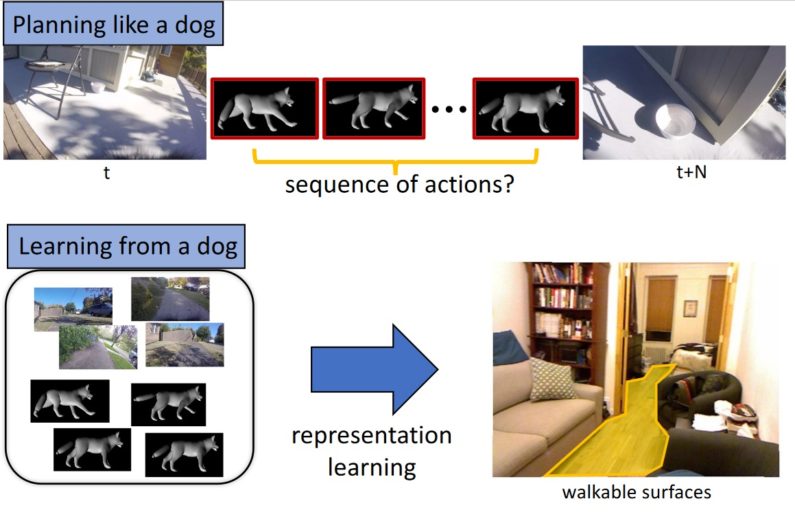
We’ve trained machine learning systems to identify objects, navigate streets and recognize facial expressions, but as difficult as they may be, they don’t even touch the level of sophistication required to simulate, for example, a dog. Well, this project aims to do just that — in a very limited way, of course. By observing the behavior of A Very Good Girl, this AI learned the rudiments of how to act like a dog.
It’s a collaboration between the University of Washington and the Allen Institute for AI, and the resulting paper will be presented at CVPR in June.
Why do this? Well, although much work has been done to simulate the sub-tasks of perception like identifying an object and picking it up, little has been done in terms of “understanding visual data to the extent that an agent can take actions and perform tasks in the visual world.” In other words, act not as the eye, but as the thing controlling the eye.
And why dogs? Because they’re intelligent agents of sufficient complexity, “yet their goals and motivations are often unknown a priori.” In other words, dogs are clearly smart, but we have no idea what they’re thinking.
As an initial foray into this line of research, the team wanted to see if by monitoring the dog closely and mapping its movements and actions to the environment it sees, they could create a system that accurately predicted those movements.
In order to do so, they loaded up a Malamute named Kelp M. Redmon with a basic suite of sensors. There’s a GoPro camera on Kelp’s head, six inertial measurement units (on the legs, tail and trunk) to tell where everything is, a microphone and an Arduino that tied the data together.
They recorded many hours of activities — walking in various environments, fetching things, playing at a dog park, eating — syncing the dog’s movements to what it saw. The result is the Dataset of Ego-Centric Actions in a Dog Environment, or DECADE, which they used to train a new AI agent.
This agent, given certain sensory input — say a view of a room or street, or a ball flying past it — was to predict what a dog would do in that situation. Not to any serious level of detail, of course — but even just figuring out how to move its body and to where is a pretty major task.
“It learns how to move the joints to walk, learns how to avoid obstacles when walking or running,” explained Hessam Bagherinezhad, one of the researchers, in an email. “It learns to run for the squirrels, follow the owner, track the flying dog toys (when playing fetch). These are some of the basic AI tasks in both computer vision and robotics that we’ve been trying to solve by collecting separate data for each task (e.g. motion planning, walkable surface, object detection, object tracking, person recognition).”
That can produce some rather complex data: For example, the dog model must know, just as the dog itself does, where it can walk when it needs to get from here to there. It can’t walk on trees, or cars, or (depending on the house) couches. So the model learns that as well, and this can be deployed separately as a computer vision model for finding out where a pet (or small legged robot) can get to in a given image.
This was just an initial experiment, the researchers say, with success but limited results. Others may consider bringing in more senses (smell is an obvious one) or seeing how a model produced from one dog (or many) generalizes to other dogs. They conclude: “We hope this work paves the way towards better understanding of visual intelligence and of the other intelligent beings that inhabit our world.”