
It’s September, which means another generation of Apple iPhones. This year, the iPhone XS (pronounced “ten ess”) adds a slightly larger sensor plus significantly more computing power via the A12 Bionic Chip to enhance the phone’s image signal processing.
But despite the ability to perform 5 trillion operations a second, the iPhone still can’t do something your dedicated ILP camera can, namely, collect as much light. And when it comes to photography, true exposure (i.e. the total number of photons captured) is directly related to image quality.
Of course, that much computing power does give some powerful workarounds.
Portrait Mode is just a (really good) mask
One of the iPhone’s new features is the ability to alter the depth-of-field post image capture (first generation portrait mode only provided one predetermined setting). The user interface employs an f-stop dial, but this is just a skeuomorphic feature that uses a full-frame aperture reference to roughly correlate the degree of depth-of-field.
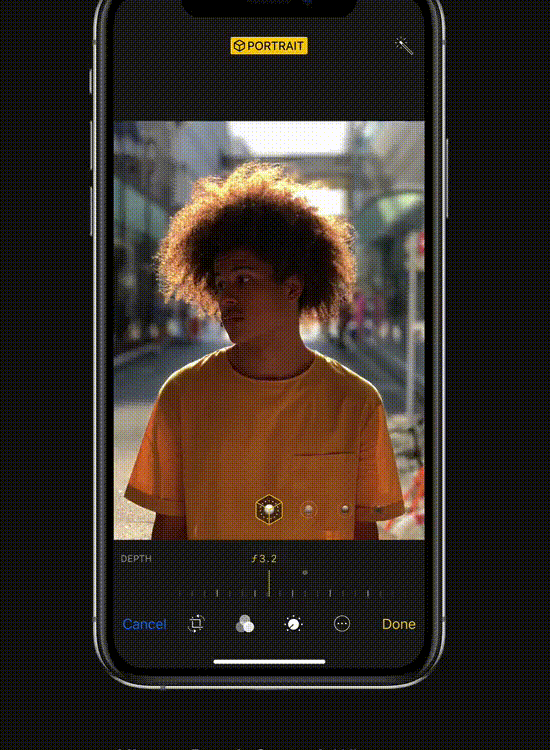
The vast majority of smartphones have fixed apertures, and the size of the sensor relative to the focal length of the lens means everything in the scene tends to be in focus. So smartphones create depth maps either using two cameras (e.g. the iPhone) or a sub-pixel design (e.g. Pixel2) combined with a neural network to determine foreground and background elements.
Even though the iPhone XS allows you to alter the depth of field in post, it’s not a light field (aka plenoptic) camera which captures both light intensity and light direction. The iPhone’s fancy processor does allow it to more intelligently mask foreground and background elements while being contextually aware of things facial features, hair, etc. The aperture dial combined with the processor means that it can increase the blur radius of elements outside the mask in real-time.
The software will undoubtedly continue to improve, but you might still run into errors that you would never get with optical capture.
Computational photography helps make excellent photos, but…
Computational photography overcomes true exposure limitations through a number of tricks including image stacking and AI. Image stacking (most commonly used for HDR) not only preserved highlights and enhances shadow detail, but it can also be used as a noise reduction strategy using median filtering.
AI brings a whole other toolbox of options to computational photography. Although the use of AI in computational photography is still nascent, we’ve already seen proof of concepts that are stunning like NVIDIA’s noise reduction and watermark removal.
Computational photography could, in theory, combine multiple photos together to get the perfect group shot where everyone is smiling (the iPhone uses neural networks for enhanced facial detection). It could also theoretically take a single image and reconstruct parts of the image to make it appear as if everyone is smiling. This type of technology presents an ethical consideration (especially for photojournalists), but it also dramatically expands the creative possibilities.
When will traditional camera manufacturers embrace a software-centric approach to photography?
Companies like Nikon, Canon, Sony, Fuji, and Hasselblad have continued to develop incredible camera systems, but they continue to focus on single exposure RAW capture for the most part. In this paradigm, toning adjustments and other image manipulation occur outside the camera. Image stacking and panoramics have to be captured as individual exposures with a manual triggering of the shutter.
But for many photographers, speed is of the essence, and the ability to load something akin to a Lightroom preset into the camera or allow for in-camera image stacking (with instantaneous noise reduction for example) would be pretty revolutionary. Standalone cameras continue to be dedicated hardware devices rather than more flexible computing machines with light capture capabilities.
It’s hard to imagine Apple making an ILP system, and equally difficult to imagine Nikon/Canon/Sony developing computational photography software and chips with onboard neural network capabilities. But just imagine the possibilities…
About the author: Allen Murabayashi is the Chairman and co-founder of PhotoShelter, which regularly publishes resources for photographers. The opinions expressed in this article are solely those of the author. Allen is a graduate of Yale University, and flosses daily. This article was also published here.